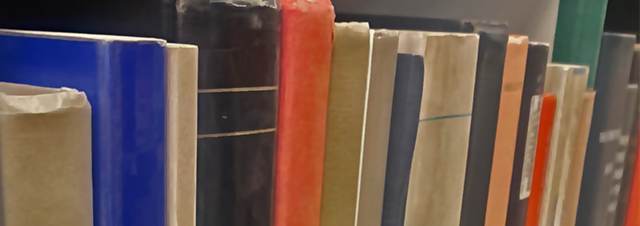
Faculty Publications
Document Type
Article
Publication Version
Published Version
Keywords
MAC layer, machine learning, sleep detection, sniffer, WiFi
Journal/Book/Conference Title
Sensors
Volume
23
Issue
14
Abstract
A good night’s sleep is of the utmost importance for the seamless execution of our cognitive capabilities. Unfortunately, the research shows that one-third of the US adult population is severely sleep deprived. With college students as our focused group, we devised a contactless, unobtrusive mechanism to detect sleep patterns, which, contrary to existing sensor-based solutions, does not require the subject to put on any sensors on the body or buy expensive sleep sensing equipment. We named this mechanism Packets-to-Predictions(P2P) because we leverage the WiFi MAC layer traffic collected in the home and university environments to predict “sleep” and “awake” periods. We first manually established that extracting such patterns is feasible, and then, we trained various machine learning models to identify these patterns automatically. We trained six machine learning models—K nearest neighbors, logistic regression, random forest classifier, support vector classifier, gradient boosting classifier, and multilayer perceptron. K nearest neighbors gave the best performance with 87% train accuracy and 83% test accuracy.
Department
Department of Computer Science
Original Publication Date
7-1-2023
Object Description
1 PDF File
DOI of published version
10.3390/s23146631
Repository
UNI ScholarWorks, Rod Library, University of Northern Iowa
Copyright
©2023 The Authors. CC BY 4.0 License
Creative Commons License
This work is licensed under a Creative Commons Attribution 4.0 International License.
Language
en
File Format
application/pdf
Recommended Citation
Jaisinghani, Dheryta and Phutela, Nishtha, "Packets-To-Prediction: An Unobtrusive Mechanism For Identifying Coarse-Grained Sleep Patterns With Wifi Mac Layer Traffic" (2023). Faculty Publications. 5416.
https://scholarworks.uni.edu/facpub/5416
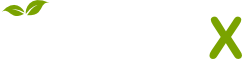
- Citations
- Citation Indexes: 2
- Usage
- Downloads: 26
- Abstract Views: 11
- Captures
- Readers: 29
- Mentions
- Blog Mentions: 1
- News Mentions: 1
Comments
First published in Sensors v.23 i.14 by MDPI. DOI: https://doi.org/10.3390/s23146631