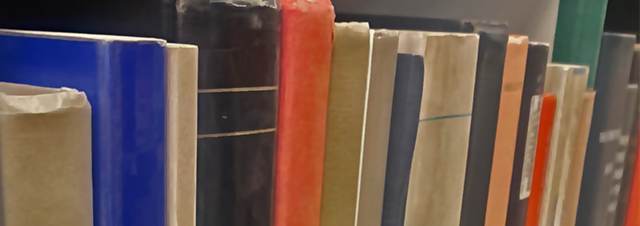
Faculty Publications
Rely: Machine Learning For Ultra-Reliable, Low-Latency Messaging In Industrial Robots
Document Type
Article
Journal/Book/Conference Title
IEEE Communications Magazine
Volume
59
Issue
4
First Page
75
Last Page
81
Abstract
Robotic factory floors are transforming the manufacturing sector by delivering an unprecedented boost to productivity. However, such a paradigm raises questions on safety and coordination, especially when in the presence of unexpected events. Time-critical communication messages for such industrial robots mandate the requirement of ultra-reliable low-latency communication (URLLC). Classical WiFi-connected industrial robots often suffer from the traditional dense network problems prevalent in production WiFi networks, where transmission of an emergency notification packet is 'best effort,' devoid of time guarantees. In this work, we propose a machine-learning-based framework called Rely that intelligently embeds the time-critical messages in the preamble of outgoing frames at the transmitter. These messages are inferred from the channel state information variations at the receiver. As Rely is implemented entirely at the physical layer, the transmitter is able to deliver information within 5 ms latency and ultra-high reliability of 99 percent. We experimentally demonstrate the feasibility of achieving URLLC with moving robots in a busy workshop with a number of other peer robots, equipment, desks, and robotic arms, as expected in a typical factory setting.
Department
Department of Computer Science
Original Publication Date
4-1-2021
DOI of published version
10.1109/MCOM.001.2000598
Repository
UNI ScholarWorks, Rod Library, University of Northern Iowa
Language
en
Recommended Citation
Sankhe, Kunal; Jaisinghani, Dheryta; and Chowdhury, Kaushik, "Rely: Machine Learning For Ultra-Reliable, Low-Latency Messaging In Industrial Robots" (2021). Faculty Publications. 81.
https://scholarworks.uni.edu/facpub/81