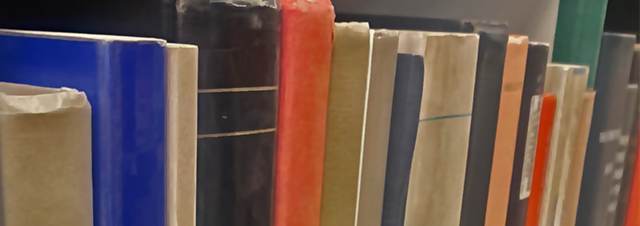
Faculty Publications
Fast Dual-Regularized Autoencoder for Sparse Biological Data
Document Type
Conference
Keywords
biological relationship inference, drug repurposing, drug target interactions, recommender systems, sparse matrix completion
Journal/Book/Conference Title
EPiC Series in Computing
Volume
101
First Page
113
Last Page
121
Abstract
Relationship inference from sparse data is an important task with applications ranging from product recommendation to drug discovery. A recently proposed linear model for sparse matrix completion has demonstrated surprising advantage in speed and accuracy over more sophisticated recommender systems algorithms. Here we extend the linear model to develop a shallow autoencoder for the dual neighborhood-regularized matrix completion problem. We demonstrate the speed and accuracy advantage of our approach over the existing state-of-the-art in predicting drug-target interactions and drug-disease associations.
Department
Department of Computer Science
Original Publication Date
7-12-2024
DOI of published version
10.29007/v896
Recommended Citation
Poleksic, Aleksandar, "Fast Dual-Regularized Autoencoder for Sparse Biological Data" (2024). Faculty Publications. 6710.
https://scholarworks.uni.edu/facpub/6710