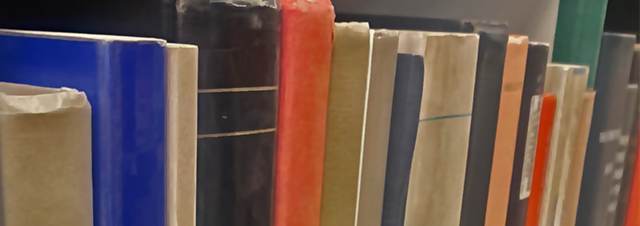
Faculty Publications
Document Type
Article
Publication Version
Published Version
Journal/Book/Conference Title
Scientific Reports
Volume
13
Issue
1
First Page
1
Last Page
11
Abstract
Past research in computational systems biology has focused more on the development and applications of advanced statistical and numerical optimization techniques and much less on understanding the geometry of the biological space. By representing biological entities as points in a low dimensional Euclidean space, state-of-the-art methods for drug-target interaction (DTI) prediction implicitly assume the flat geometry of the biological space. In contrast, recent theoretical studies suggest that biological systems exhibit tree-like topology with a high degree of clustering. As a consequence, embedding a biological system in a flat space leads to distortion of distances between biological objects. Here, we present a novel matrix factorization methodology for drug-target interaction prediction that uses hyperbolic space as the latent biological space. When benchmarked against classical, Euclidean methods, hyperbolic matrix factorization exhibits superior accuracy while lowering embedding dimension by an order of magnitude. We see this as additional evidence that the hyperbolic geometry underpins large biological networks.
Department
Department of Computer Science
Original Publication Date
12-1-2023
DOI of published version
10.1038/s41598-023-27995-5
Repository
UNI ScholarWorks, Rod Library, University of Northern Iowa
Copyright
©2023 Aleksandar Poleksic. This is an open access article distributed under the terms of the Creative Commons Attribution License, which permits unrestricted use, distribution, and reproduction in any medium, provided the original author and source are credited.
Creative Commons License
This work is licensed under a Creative Commons Attribution 4.0 International License.
Language
en
File Format
application/pdf
Recommended Citation
Poleksic, Aleksandar, "Hyperbolic Matrix Factorization Improves Prediction Of Drug-Target Associations" (2023). Faculty Publications. 5354.
https://scholarworks.uni.edu/facpub/5354
Comments
First published in Scientific Reports, 13, 959 (2023) published by Nature Portfolio. DOI: https://doi.org/10.1038/s41598-023-27995-5