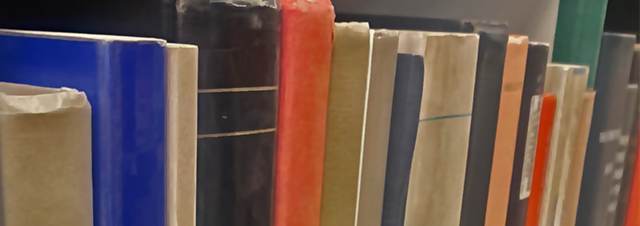
Faculty Publications
Ev Fleet Charging Load Forecasting Based On Multiple Decomposition With Ceemdan And Swarm Decomposition
Document Type
Article
Keywords
CEEMDAN, electric vehicle, fleet charging, forecasting, signal decomposition, swarm decomposition
Journal/Book/Conference Title
IEEE Access
Volume
10
First Page
62330
Last Page
62340
Abstract
As the transition to electric mobility is accelerating, EV fleet charging loads are expected to become increasingly significant for power systems. Hence, EV fleet load forecasting is vital to maintaining the reliability and safe operation of the power system. This paper presents a new multiple decomposition based hybrid forecasting model for EV fleet charging. The proposed approach incorporates the Swarm Decomposition (SWD) into the Complete Ensemble Empirical Mode Decomposition Adaptive Noise (CEEMDAN) method. The multiple decomposition approach offers more stable, stationary, and regular features of the original signals. Each decomposed signal is fed into artificial intelligence based forecasting models including multi-layer perceptron (MLP), long short-term memory (LSTM) and bidirectional LSTM (Bi-LSTM). Real EV fleet charging data sets from the field are used to validate the performance of the models. Various statistical metrics are used to quantify the prediction performance of the proposed model through a comparative analysis of the implemented models. It is demonstrated that the multiple decomposition approach improved the model performance with an R2 value increasing from 0.8564 to 0.9766 as compared to the models with single decomposition.
Department
Department of Applied Engineering and Technical Management
Original Publication Date
1-1-2022
DOI of published version
10.1109/ACCESS.2022.3182499
Recommended Citation
Dokur, Emrah; Erdogan, Nuh; and Kucuksari, Sadik, "Ev Fleet Charging Load Forecasting Based On Multiple Decomposition With Ceemdan And Swarm Decomposition" (2022). Faculty Publications. 5344.
https://scholarworks.uni.edu/facpub/5344