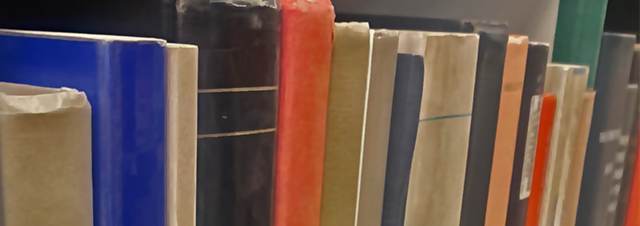
Faculty Publications
Quantifying Below-Water Fluvial Geomorphic Change: The Implications Of Refraction Correction, Water Surface Elevations, And Spatially Variable Error
Document Type
Article
Keywords
Change detection, Fluvial, Geomorphology, Machine learning, Refraction correction, Remotely piloted aircraft system, Structure-from-motion photogrammetry, Topographic error, Water surface elevation
Journal/Book/Conference Title
Remote Sensing
Volume
11
Issue
20
Abstract
Much of the geomorphic work of rivers occurs underwater. As a result, high resolution quantification of geomorphic change in these submerged areas is important. Currently, to quantify this change, multiple methods are required to get high resolution data for both the exposed and submerged areas. Remote sensing methods are often limited to the exposed areas due to the challenges imposed by the water, and those remote sensing methods for below the water surface require the collection of extensive calibration data in-channel, which is time-consuming, labour-intensive, and sometimes prohibitive in difficult-to-access areas. Within this paper, we pioneer a novel approach for quantifying above- and below-water geomorphic change using Structure-from-Motion photogrammetry and investigate the implications of water surface elevations, refraction correction measures, and the spatial variability of topographic errors. We use two epochs of imagery from a site on the River Teme, Herefordshire, UK, collected using a remotely piloted aircraft system (RPAS) and processed using Structure-from-Motion (SfM) photogrammetry. For the first time, we show that: (1) Quantification of submerged geomorphic change to levels of accuracy commensurate with exposed areas is possible without the need for calibration data or a different method from exposed areas; (2) there is minimal difference in results produced by different refraction correction procedures using predominantly nadir imagery (small angle vs. multi-view), allowing users a choice of software packages/processing complexity; (3) improvements to our estimations of water surface elevations are critical for accurate topographic estimation in submerged areas and can reduce mean elevation error by up to 73%; and (4) we can use machine learning, in the form of multiple linear regressions, and a Gaussian Naïve Bayes classifier, based on the relationship between error and 11 independent variables, to generate a high resolution, spatially continuous model of geomorphic change in submerged areas, constrained by spatially variable error estimates. Our multiple regression model is capable of explaining up to 54% of magnitude and direction of topographic error, with accuracies of less than 0.04 m. With on-going testing and improvements, this machine learning approach has potential for routine application in spatially variable error estimation within the RPAS-SfM workflow.
Department
Department of Geography
Original Publication Date
10-1-2019
DOI of published version
10.3390/rs11202415
Repository
UNI ScholarWorks, Rod Library, University of Northern Iowa
Language
en
Recommended Citation
Woodget, Amy S.; Dietrich, James T.; and Wilson, Robin T., "Quantifying Below-Water Fluvial Geomorphic Change: The Implications Of Refraction Correction, Water Surface Elevations, And Spatially Variable Error" (2019). Faculty Publications. 462.
https://scholarworks.uni.edu/facpub/462