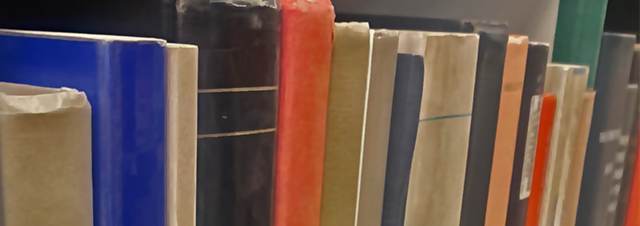
Faculty Publications
Sequence-Dependent Clustering Of Parts And Machines: A Fuzzy Art Neural Network Approach
Document Type
Article
Journal/Book/Conference Title
International Journal of Production Research
Volume
37
Issue
12
First Page
2793
Last Page
2816
Abstract
This study addresses the problem of identifying families of parts having a similar sequence of operations. This is a prerequisite for the implementation of cellular manufacturing, group technology, just-in-time manufacturing systems, and for streamlining material flows in general. A pattern recognition approach based on artificial neural networks is proposed, and it is shown that the Fuzzy ART neural network can be effectively utilized for this application. First, a representation scheme for operation sequences is developed, followed by an illustrative example. A more comprehensive experimental verification, based on the mixture-model approach is then performed to evaluate its performance. The experimental factors include size of the part-machine matrix, proportion of voids, proportion of exceptional elements, and vigilance threshold. It is shown that this neural network is effective in identifying good clustering solutions, consistently and with relatively fast execution times. © 1999 Taylor & Francis Group, LLC.
Department
Department of Management
Original Publication Date
1-1-1999
DOI of published version
10.1080/002075499190527
Recommended Citation
Suresh, N. C.; Slomp, J.; and Kaparthi, S., "Sequence-Dependent Clustering Of Parts And Machines: A Fuzzy Art Neural Network Approach" (1999). Faculty Publications. 3823.
https://scholarworks.uni.edu/facpub/3823