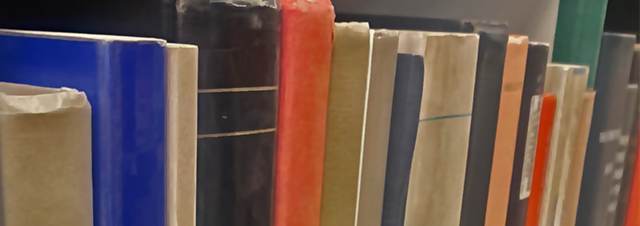
Faculty Publications
Bayesian Modeling And Inference For Geometrically Anisotropic Spatial Data
Document Type
Article
Keywords
Contour plot, Correlation functions, Importance sampling, Second-order stationary, Semivariogram, Wishart distribution
Journal/Book/Conference Title
Mathematical Geology
Volume
31
Issue
1
First Page
67
Last Page
83
Abstract
A geometrically anisotropic spatial process can be viewed as being a linear transformation of an isotropic spatial process. Customary semivariogram estimation techniques often involve ed hoc selection of the linear transformation to reduce the region to isotropy and then fitting a valid parametric semivariogram to the data under the transformed coordinates. We propose a Bayesian methodology which simultaneously estimates the linear transformation and the other semivariogram parameters. In addition, the Bayesian paradigm allows full inference for any characteristic of the geometrically anisotropic model rather than merely providing a point estimate. Our work is motivated by a dataset of scallop catches in the Atlantic Ocean in 1990 and also in 1993. The 1990 data provide useful prior information about the nature of the anisotropy of the process. Exploratory data analysis (EDA) techniques such as directional empirical semivariograms and the rose diagram are widely used by practitioners. We recommend a suitable contour plot to detect departures from isotropy. We then present a fully Bayesian analysis of the 1993 scallop data, demonstrating the range of inferential possibilities.
Department
Department of Mathematics
Original Publication Date
1-1-1999
DOI of published version
10.1023/A:1007593314277
Recommended Citation
Ecker, Mark D. and Gelfand, Alan E., "Bayesian Modeling And Inference For Geometrically Anisotropic Spatial Data" (1999). Faculty Publications. 3813.
https://scholarworks.uni.edu/facpub/3813