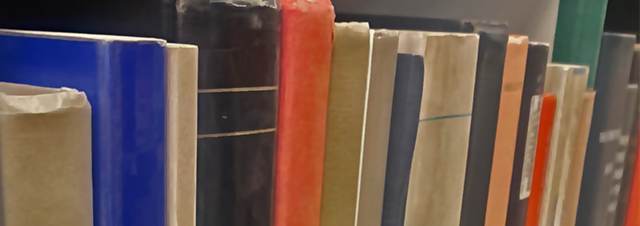
Faculty Publications
Designing Predictive Maintenance Systems Using Decision Tree-Based Machine Learning Techniques
Document Type
Article
Keywords
Classification techniques, Machine learning, Maintenance, Predictive maintenance systems, Reliability
Journal/Book/Conference Title
International Journal of Quality and Reliability Management
Volume
37
Issue
4
First Page
659
Last Page
686
Abstract
Purpose: The after-sale service industry is estimated to contribute over 8 percent to the US GDP. For use in this considerably large service management industry, this article provides verification in the application of decision tree-based machine learning algorithms for optimal maintenance decision-making. The motivation for this research arose from discussions held with a large agricultural equipment manufacturing company interested in increasing the uptime of their expensive machinery and in helping their dealer network. Design/methodology/approach: We propose a general strategy for the design of predictive maintenance systems using machine learning techniques. Then, we present a case study where multiple machine learning algorithms are applied to a particular example situation for an illustration of the proposed strategy and evaluation of its performance. Findings: We found progressive improvements using such machine learning techniques in terms of accuracy in predictions of failure, demonstrating that the proposed strategy is successful. Research limitations/implications: This approach is scalable to a wide variety of applications to aid in failure prediction. These approaches are generalizable to many systems irrespective of the underlying physics. Even though we focus on decision tree-based machine learning techniques in this study, the general design strategy proposed can be used with all other supervised learning techniques like neural networks, boosting algorithms, support vector machines, and statistical methods. Practical implications: This approach is applicable to many different types of systems that require maintenance and repair decision-making. A case is provided for a cloud data storage provider. The methods described in the case can be used in any number of systems and industrial applications, making this a very scalable case for industry practitioners. This scalability is possible as the machine learning techniques learn the correspondence between machine conditions and outcome state irrespective of the underlying physics governing the systems. Social implications: Sustainable systems and operations require allocating and utilizing resources efficiently and effectively. This approach can help asset managers decide how to sustainably allocate resources by increasing uptime and utilization for expensive equipment. Originality/value: This is a novel application and case study for decision tree-based machine learning that will aid researchers in developing tools and techniques in this area as well as those working in the artificial intelligence and service management space.
Department
Department of Management
Original Publication Date
3-30-2020
DOI of published version
10.1108/IJQRM-04-2019-0131
Repository
UNI ScholarWorks, Rod Library, University of Northern Iowa
Language
en
Recommended Citation
Kaparthi, Shashidhar and Bumblauskas, Daniel, "Designing Predictive Maintenance Systems Using Decision Tree-Based Machine Learning Techniques" (2020). Faculty Publications. 334.
https://scholarworks.uni.edu/facpub/334