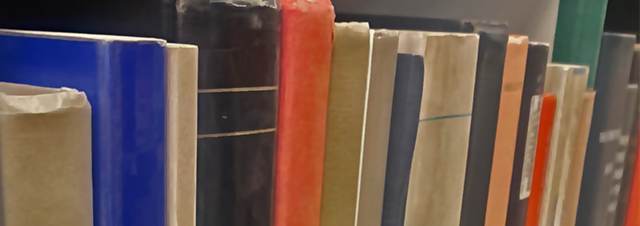
Faculty Publications
Object-Oriented Classification Of Lidar-Fused Hyperspectral Imagery For Tree Species Identification In An Urban Environment
Document Type
Conference
Journal/Book/Conference Title
2007 Urban Remote Sensing Joint Event, URS
Abstract
The objective of the current study was to develop a methodology for the identification of tree species in an urban environment by using Quickbird multispectral data, AISA hyperspectral data, AISA Eagle hyperspectral data and Leica ALS50 LiDAR data. For this research, object-oriented classification was performed using eCognition Professional. The classifications were performed on each of the images available with and without the aid of LiDAR. Elevation and intensity data was used to create images segments as well as user-defined class rules. Classes included honey locust, white pine, crab apple, sugar maple, white spruce, American basswood, pin oak and ash. Initial results indicate fusing LiDAR data with these imageries showed an increase in overall classification accuracy for all datasets. Increases in overall accuracy ranged from 12 to 24 percent over classifications based on spectral imagery alone. There were some more substantial increases in some individual species accuracies, particularly classes that consisted of smaller objects such as saplings or shrubbery. © 2007 IEEE.
Department
Department of Geography
Original Publication Date
9-28-2007
DOI of published version
10.1109/URS.2007.371845
Recommended Citation
Sugumaran, Ramanathan and Voss, Matthew, "Object-Oriented Classification Of Lidar-Fused Hyperspectral Imagery For Tree Species Identification In An Urban Environment" (2007). Faculty Publications. 2560.
https://scholarworks.uni.edu/facpub/2560